Unveiling Hidden Patterns: How Box Plots Can Uncover Outliers and Anomalies 10/16
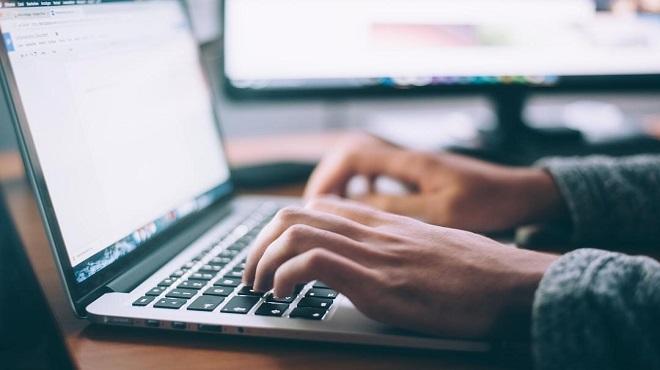
Box plots, also known as whisker plots, have long been used by statisticians and data analysts to visualize the distribution of data. Though seemingly simplistic, these graphical representations are a powerful tool for deciphering trends and patterns, detecting outliers, and identifying data anomalies. This article delves into the depths of box plots—unraveling their construction, understanding their interpretation, and unveiling the hidden patterns they reveal. Keep reading to learn about their applications and benefits, and how your organization can leverage these unassuming visuals to glean valuable insights into your data.
Understanding the Basics of Box Plots
A box plot, or whisker plot, epitomizes statistical representation. It simplifies data comparison by presenting a visual summary of distribution parameters—the median, quartiles, and potential outliers. Rooted in descriptive statistics, each box plot is an amalgamation of a “box” and a pair of “whiskers.” The box covers the interquartile range, encompassing the middle 50 percent of observations, with a line indicating the median (i.e., the second quartile or the 50th percentile). The whiskers, extending from the box, point out the plausible range for the remainder of the data. A more detailed explanation of this visualizing tool can be found in this box plot article.
Suppose a set of observations ranges from the lowest value, referred to as the minimum, to the highest value, known as the maximum. These are notated as Q1 and Q3, respectively, with Q2 marking the median. This terminology is universally recognized by statisticians and helps in comparability between data sets.
In order to understand and reproduce a box plot, it is important to understand quartiles (Q1, Q2, and Q3), median(m), and potential outliers. These statistical terms allow one to get a bird’s eye view of the data and make meaningful comparisons.
Leveraging Box Plots for Comparative Analysis
While a single box plot can provide a glimpse of your data distribution, the true power of these visualizations emerges when using them for comparative analysis. By juxtaposing multiple box plots on a single axis, it’s possible to compare various datasets side by side, to analyze trends and patterns, geographical variations, or changes over time.
In market research, for example, box plots can be used to compare customer satisfaction ratings across different products or services. Similarly, in healthcare, box plots can illustrate patient recovery times across different treatment modalities. This level of comparative analysis can reveal patterns that may not have been evident when viewing the data individually.
In the realm of data analytics, these standalone graphics become powerful decision-making tools when used collectively. The comparative insight they offer into data distribution makes them valuable for a diverse spectrum of fields, from business and economics to environmental science and healthcare.
Using Box Plots to Identify Outliers and Anomalies
Outliers are observations that are significantly different from others. They can disrupt the normal distribution of data and skew the overall results, making it difficult to draw accurate conclusions. Box plots offer an intuitive way to identify these outliers.
The whiskers of the box plot extend to the minimum and maximum data points within the 1.5 interquartile range on either side of the box. Any data points falling outside of these whiskers are considered potential outliers and are typically marked with an asterisk or a circle.
Box plots’ ability to visually identify outliers and anomalies adds a significant value to data analysis. Recognizing these outliers can lead to more precise interpretation of data, adjustment of strategies, or even uncovering of data errors or fraudulent activity. This function makes box plots a reliable tool for data quality assurance checks and for ensuring statistical validity.
Applying Box Plots in Various Fields
Box plots are not confined to any one specific field or industry. On the contrary, their universal applicability makes them a popular choice for various disciplines. From finance and economics, where they have been employed to track stock prices and economic data, to biology and medicine, where they have been used to compare gene expression levels and patient recovery times, box plots have found widespread use.
In education, box plots can illuminate the spread of grades or test scores, helping educators assess their students’ performance. In market research, box plots illustrate customer attitudes, enabling companies to identify trends and determine their products’ success.
Overall, box plots serve as a reliable tool for visual data exploration and analysis, providing insightful representations to understand and compare data distributions, detect outliers, and carry out descriptive or predictive analytics. Despite some limitations, they continue to be a preferred method for many statisticians, data scientists, and analysts.